Harnessing the Transformative
Becoming interested in artificial intelligence (AI) during his first year of graduate school at Drexel University, Dr. Vasant Honavar was encouraged by an advisor to find a university with a strong graduate program in AI. This led him to the University of Wisconsin where he was able to take graduate courses in computer science, electrical engineering, psychology and neuroscience while pursuing research in AI under the mentorship of Professor Leonard Uhr, one of the early pioneers in AI. Dr. Honavar earned a master’s degree in computer science, and later his Ph.D., with his thesis focusing on neural network algorithms for machine learning.
Fast forward to today and Dr. Honavar is now Huck Chair in Biomedical Data Sciences and Artificial Intelligence, Founding Director, Center for Artificial Intelligence Foundations and Scientific Applications (CENSAI), and the Center for Big Data Analytics and Discovery Informatics at the Pennsylvania State University. In addition, Honavar is a professor of Data Sciences in the College of Information Sciences and Technology (IST), with graduate faculty appointments in Informatics, Computer Science and Engineering, Bioinformatics and Genomics, Neuroscience, Public Health Sciences, and Operations Research. “My core interest has always been AI, with a primary research focus on machine learning and knowledge representation and inference,” says Honavar. Over the years, Honavar has developed scalable methods for learning predictive models from (distributed, heterogeneous, multi-modal, longitudinal, and very high-dimensional) big data; deep learning methods for representation learning from complex data; eliciting causal effects from observational and experimental data; selective sharing of knowledge across disparate knowledge bases; and applications in bioinformatics (characterization and prediction of protein-protein, protein-RNA interactions, interfaces, and complexes; prediction of B-cell and T-cell epitopes; eliciting disease biomarkers from multi-omics data) and health informatics (predictive and causal modeling of health risks and health outcomes from clinical, socio-demographic, and behavioral data).
“A major focus of my work has been on looking at large data sets, and building predictive models,” says Honavar. “I also work on causal inference, which goes beyond making predictions to identify what interventions can be done to change the outcomes. This is very important for healthcare, for example. Instead of just telling a person they have an elevated risk of heart disease, causal inference reveals what can be done to reduce that risk.”
Honavar’s current work on knowledge representation focuses on on representing and reasoning about complex preferences of individuals. “A key goal of this work is to develop languages and tools that will allow multiple stakeholders to express their preferences over alternatives, e.g., in healthcare, public policy, etc. that can be used to identify those that optimally conform to stakeholder preferences,“ says Honavar. His current work on machine learning focuses on building accurate, explainable, predictive models from complex longitudinal data, with applications to predictive modeling of health risks and health outcomes. After joining Penn State, I’ve been working with electronic health records data and trying to predict health risks and outcomes, as well as identifying health disparities.”
“While advances in machine learning (ML) have dramatically accelerated some of the key steps in scientific discovery, particularly data acquisition and model fitting, the rest of the key elements of the scientific process remain largely untouched and untamed by artificial intelligence (AI). Addressing this grand challenge requires concerted advances across all areas of AI. Currently, our three focus areas are life sciences, material science, and healthcare.”
— Vasant Honavar, Ph.D.
Huck Chair in Biomedical Data Sciences and Artificial Intelligence,
Founding Director, Center for Artificial Intelligence Foundations and Scientific Applications (CENSAI), and
the Center for Big Data Analytics and Discovery Informatics, Pennsylvania State University
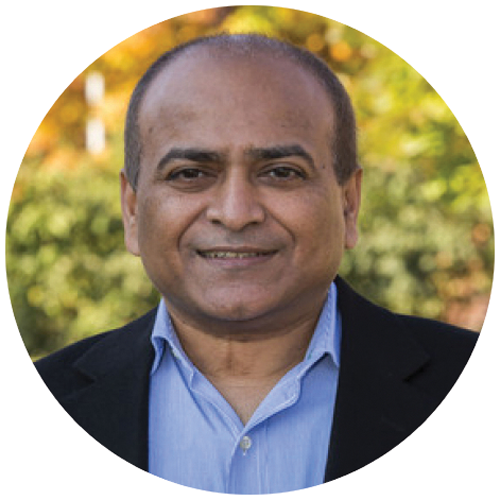
Advancing AI through Multi-institutional Collaboration
The Center for Artificial Intelligence Foundations and Scientific Applications (CENSAI) brings together more than 40 researchers from across multiple colleges and disciplines across Penn State with the goal of advancing AI to dramatically accelerate scientific discovery. “While advances in machine learning (ML) have dramatically accelerated some of the key steps in scientific discovery, particularly data acquisition and model fitting, the rest of the key elements of the scientific process remain largely untouched and untamed by artificial intelligence (AI) and constitute major bottlenecks to scientific progress,” says Honavar. “These include generating hypotheses; prioritizing, optimizing and executing experiments; integrating data, models, and simulations across disparate data modalities and scales; drawing inferences and constructing explanations; organizing and orchestrating collaboration; and synthesizing knowledge across disciplines,” he adds. According to Honavar, accelerating science presents a grand challenge for AI. “Addressing this grand challenge requires concerted advances across all areas of AI. CENSAI is organized around addressing this grand challenge. Currently, our three focus areas are life sciences, material science, and healthcare,” says Honavar.
When it comes to research where foundational and applied AI converge, Honavar says interdisciplinary collaboration is essential, “The formation of teams with the right combination of expertise and interests is crucial for successfully advancing AI, and enabling scientific discoveries in different domains using the power of AI. We’re creating research groups and infrastructure to support them that didn’t exist before and it’s allowing our researchers to develop, participate in, and when appropriate, lead, multi-institutional collaborations. CENSAI has already had successes in life sciences, material sciences, and health sciences.”
“I expect AI will have an impact across all fields, especially in areas where complex data is generated,” continues Honavar. “This technology will also have a role in education, but I envision it being used to create personalized learning experiences, rather than just using tools like ChatGPT in the classroom. I picture interactive systems that generate problems based on each student’s progress and can respond with customized interactions. Instead of replacing instructors as some may fear, I see AI as supplementing course content to improve education.”
The Importance of Regulating AI
Just like any technological advance, Honavar says AI can have both positive and negative consequences. “With the introduction of fire, for example, we could cook food and stay warm, but it could also be very destructive if left uncontrolled. AI is similar in that it has the potential to have a positive impact if deployed in a socially responsible way, but it could also cause substantial harm if misused, e.g., to spread misinformation. Another analogy is the industrial revolution when automated production became available and many were displaced from their jobs. While the fruits of the industrial revolution were beneficial to our society, it was not a painless process. Similarly, new technologies may automate certain job tasks, but also generate positions that didn’t exist before. We will need broad-based training in AI, as well as policies that ensure that the societal benefits of AI are maximized while its potential for harm are minimized.”
Although early work on artificial intelligence dates back to the 1950s, it is only in the past decade or so that AI has developed to a point where AI applications are ubiquitous. “The transition of AI from an academic research enterprise to industrial practice was enabled by a confluence of unprecedented availability of massive data sets, compute power, together with advances in machine learning,” says Honavar. “Developments like the large language models and GPT are in many ways very fascinating, and have brought AI to the masses but there are many difficult challenges – some technical, and some socio-technical, that remain to be addressed. Going forward, we must learn ways to harness AI that will help improve society and enrich our lives.”
The Emergence of Quantum Computing
Penn State, like many other institutions, has been looking into how to build research capacity in quantum computing. There are research groups at Penn State exploring quantum materials, quantum information science, and quantum computing applications, e.g., in machine learning and combinatorial drug discovery. Unlike classical computers, quantum computing devices use quantum bits called qubits. “Much of the work in quantum computing is currently done using simulators or machines with dozens of qubits,” explains Honavar. “I think in the next ten years, we will have machines with enough qubits to handle useful calculations. I do not see quantum computing replacing classical computing, but rather augmenting classical systems for certain types of problems. In the near to medium term, classical-quantum hybrid systems seem quite promising to explore.”
“The biggest challenge we have at the moment is not everyone has access to quantum computers,” continues Honavar. “Organizations like the NSF are funding centers or consortia that offer access to scarce quantum computing resources for research and education. With quantum computation, you must think about problems very differently and this will require training people in how to effectively formulate problems in ways that can take advantage of quantum computing capabilities. I think advances in quantum computing will contribute to advances in AI and vice versa. It will be exciting to see what new capabilities emerge from the interplay between quantum computing and AI the future.”
“I expect AI will have an impact across all fields, especially in areas where complex data is generated. This technology will also have a role in education, but I envision it being used to create personalized learning experiences, rather than just using tools like ChatGPT in the classroom. I picture interactive systems that generate problems based on each student’s progress and can respond with customized interactions. Instead of replacing instructors as some may fear, I see AI as supplementing course content to improve education.”
— Vasant Honavar, Ph.D.
Huck Chair in Biomedical Data Sciences and Artificial Intelligence,
Founding Director, Center for Artificial Intelligence Foundations and Scientific Applications (CENSAI), and
the Center for Big Data Analytics and Discovery Informatics, Pennsylvania State University
Building Community Engagement and Outreach
Edge has a long history of multi-year collaborations with Penn State ranging from the NSF-funded Virtual Data Collaboratory project to the Community Research Infrastructure for Integrated AI-Enabled Malware and Network Data Analytics. “The University’s collaborative environment fosters interdisciplinary research, allowing for the exchange of ideas and the potential for groundbreaking discoveries,” says Dr. Forough Ghahramani, Assistant Vice President for Research, Innovation, and Sponsored Programs for Edge. “Additionally, Penn State has a strong commitment to community engagement and outreach, which provides opportunities for impactful research collaborations that address real-world challenges.”
“Dr. Ghahramani has been instrumental in the outreach and education components of many of the projects we are doing,” adds Honavar. “Having the infrastructure and connections in place allows somebody like me, for example, to develop and package the content and make it accessible to a much wider audience, including K-12, community colleges, and under-resourced institutions. Creating a talent pipeline is often a big challenge in STEM and in computing; where women and minority groups are often underrepresented. We need more talent across all fields. With the technologies we are developing and the potential impact on society, having diverse experiences and perspectives at the table is essential. I believe regional networks, like Edge, play an important role in supporting that pipeline.”
Understanding the Fundamentals of AI
Preparing the workforce with the skills and knowledge necessary to meet societal needs of today and the future continues to be a key focus for the education and research community. “Throughout the whole spectrum of research there is an important societal challenge that is being addressed through research; with the results being translated into impact,” says Honavar. “When you look at AI, the workforce requirements range from getting people interested and trained in AI so they can contribute to applications of AI in research, to technologists who can deploy AI across a broad range of applications, to engineers who can build the systems, all the way to leaders and decision-makers who need to understand both the benefits and potential harms of AI to develop policies that maximize the societal benefits of AI while minimizing its harm. Today, we see computing, AI and data science touching virtually all aspects of human lives, which will hopefully make opportunities to work with advanced technology more accessible to a larger group of people.”
To use AI successfully and responsibly, Honavar says the focus should not just be on computer science, but also on our societal values. “We must first educate individuals on what these technologies are capable of and the consequences of their proper and improper uses. Penn State is creating a general education course on AI without programming prerequisites that any student can take. I think this level of introduction should also migrate down to K-12 to begin that exploration early. Not all individuals are going to be building AI systems necessarily, but they will be impacted by AI and should be prepared to take an informed stance on what is socially responsible.”
“AI got me into computing, and it is a field I know and love,” continues Honavar. “The history of AI is intimately tied to the history of computing. The working hypothesis of AI has been that computing offers for AI, what calculus offered for physics. From the very beginning, AI has had multiple aims. One is to answer the purely scientific question of understanding intelligence (natural or artificial?). A second goal of AI is to explore the entire design space of these systems, with human intelligence being one point in that space. A third goal of AI is to build intelligent artifacts for specific applications by automating tasks requiring intelligence. A fourth goal of AI is to augment and extend human intellect and abilities. Not everyone in the field shares the same goals. For that matter, even the same people may have different goals in different aspects of their work in AI. Debates about regulating AI etc. have to distinguish between AI research versus AI applications. Research is indispensable for understanding the capabilities and limits of AI and for developing better AI technologies. Constraining basic research in AI can be counterproductive. On the other hand, some regulation seems necessary for responsible deployment of AI technologies in high stakes applications.”
“AI has very interdisciplinary roots and those who first explored the topic had very broad training, including psychology, philosophy, and mathematics. Over the years, training has often narrowed and become more specialized, and we’ve lost some of the ethos of understanding intelligence. To determine what defines responsible use of AI and clearly identify the benefits and risks—as well as harness the opportunities—we must understand the foundational elements. By using this base knowledge, we can keep pace with this rapidly growing field and unlock AI’s potential in a way that will positively impact our society.”